Incrementality tests are a method of measuring the effectiveness of marketing campaigns. They involve comparing the results of a campaign with those of a control group to determine the actual impact of the campaign. This approach allows for a more precise evaluation of a campaign’s effectiveness compared to traditional methods, which simply measure overall performance without accounting for external factors.
Incrementality tests are particularly useful for measuring the effectiveness of digital marketing campaigns, where it is often difficult to determine the actual impact of a campaign due to the complexity of interactions between different channels and halo effects. By comparing the results of a campaign with those of a control group, marketers can isolate the direct impact of the campaign and assess its real contribution to the company’s objectives.
However, in practice, it can be challenging to implement effective incrementality tests. Here are some common challenges marketers face when trying to measure the incrementality of their campaigns:
-
Defining Objectives : Before setting up an incrementality test, it is essential to clearly define the campaign’s objectives and the metrics to be measured. This ensures that the test is well-designed to address the specific questions the business has. Often, marketers struggle to define clear objectives and end up measuring the wrong metrics, leading to inaccurate results.
-
Selecting the Control Group : Choosing the control group is crucial for ensuring the validity of the test results. It is important to select a control group that is representative of the target population and ensure that it is comparable to the group exposed to the campaign. Generally, businesses only have access to aggregated data, such as total sales, which can make creating an equivalent control group difficult.
-
Measuring Incrementality : Once the test is set up, it is crucial to correctly measure the campaign’s incrementality. This involves comparing the results of the group exposed to the campaign with those of the control group and isolating the direct impact of the campaign by accounting for external factors. The difficulty often lies in quantifying this impact and separating the effects of the campaign from other factors that may influence the results.
-
Interpreting Results : Finally, once the test results are obtained, it is essential to interpret them correctly to draw relevant conclusions. This often involves rigor in data analysis and consideration of the limitations and potential biases of the test. Biases are numerous, and it is often difficult to identify and correct them. It is important to remain critical and not draw hasty conclusions from the results of a single test. It is often necessary to conduct multiple tests to confirm the conclusions and ensure their validity. Failure should be considered a possibility, not a fatality. Many clients expect perfect tests, but this is almost never the case. It is important to communicate well about the limitations of the tests and not to overpromise.
Types of Incrementality Tests
There are several types of incrementality tests, each suited to specific objectives and contexts. Here are some of the most common types of tests:
-
Randomized Controlled Trials (RCTs) : Also known as A/B tests, this method involves randomly dividing the audience into control and test groups. The test group is exposed to the marketing campaign, while the control group is not. The difference in results between the two groups is attributed to the campaign. In practice, it is often difficult to set up rigorous RCTs due to operational constraints and potential biases.
-
Geography-Based Tests : This approach involves selecting different geographical regions to receive different marketing treatments or no treatment at all. By comparing performance indicators (such as sales or traffic) across these regions, marketers can deduce the impact of their campaigns. The problem is that regions are not always comparable, and halo effects can bias the results.
-
Time-Based Tests : Marketers can measure incrementality by altering exposure to a campaign over different periods. For example, comparing sales during a campaign period to a period without campaign activities can highlight the incremental impact of the campaign. However, this method does not account for seasonal factors or halo effects. Therefore, caution must be exercised in interpreting the results or finding another way to account for these effects.
-
Switchback Tests : Similar to time-based tests, switchback tests involve alternating periods of exposure and non-exposure to a marketing initiative. This method is particularly useful in environments where external factors are minimally controlled, helping to average out variability over time. In practice, these tests are often difficult to implement due to operational constraints. It’s hard to tell a marketer to stop a campaign to see if it’s effective, especially in an ‘always on’ advertising context.
-
Algorithmic Attribution Models : These models use advanced statistical techniques to attribute credit to various marketing touchpoints along a customer’s path to purchase. By analyzing changes in results with varying levels of exposure to different channels, these models estimate incremental impacts. However, these models are often complex and require granular data to function properly. They can also be sensitive to biases and modeling errors.
-
Matched Market Tests : In this approach, markets are matched based on similar characteristics, with one market receiving the campaign and the other serving as a control. The comparison of results between these paired markets can provide insights into the incrementality of the campaign. Solutions like Meta’s ‘geolift’ can help set up these tests, but they often require significant data volumes to be meaningful. It is often difficult to find matched markets, and halo effects can bias the results.
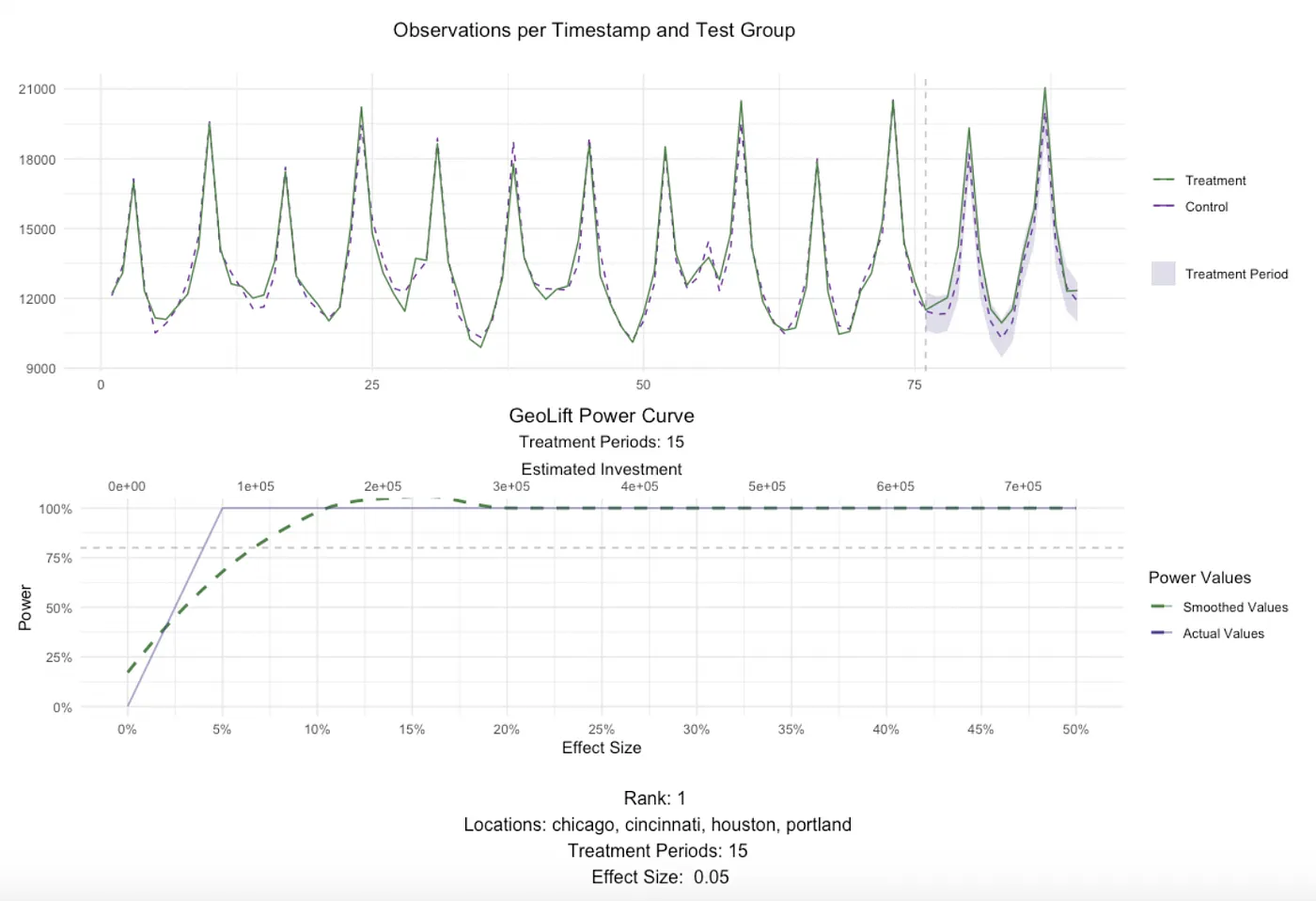
Matched Market Tests using Geolift by META
Incrementality in ‘Always On’ Advertising
In a context of ‘always on’ advertising, where marketing campaigns are continuously broadcast, it can be difficult to measure incrementality accurately. Halo effects, complex interactions between different channels, and external factors can bias the results of incrementality tests.
It is important to keep in mind that incrementality tests are not a panacea and have limitations. It is essential to consider these limitations when designing and interpreting tests to avoid erroneous conclusions.
For successful tests, it is recommended, when possible, to have a ‘washout’ period before and after the campaign to ensure that the effects of the campaign are well isolated. The ‘washout’ period is a time during which no campaigns are broadcast, allowing the effects of the previous campaign to be measured and ensuring that the effects of the current campaign are well isolated. This reduces halo effects and yields more accurate results. This is particularly important in an ‘always on’ advertising context, where halo effects can be significant. But let’s be clear, few companies can afford not to broadcast campaigns for an extended period. It is therefore essential to find a balance between the accuracy of the tests and the continuity of the campaigns.
It is also recommended to have multiple lift evaluation techniques, from the simplest to the most complex, to confirm the results and ensure their validity. Incrementality tests are not an exact science, and it is important to consider the limitations and potential biases to obtain reliable results. The simplest method involves naively comparing sales from the group exposed to the campaign with those from the control group, or the evolution of sales before and after the campaign, or the evolution of sales versus a reference period (last year, same period, etc.). While keeping in mind that these simple methods do not account for halo effects and complex interactions between different channels.
The Future of Incrementality Tests
When I was an engineer in the oil industry, I worked on reservoir modeling. We used numerical resolution methods to simulate fluid behavior in reservoirs and optimize production. Incrementality tests in marketing remind me of these modeling methods. We are trying to understand the impact of a marketing campaign by isolating the effects of the campaign from other factors that may influence the results. It is a complex challenge, but essential for measuring the effectiveness of campaigns and allocating marketing resources efficiently.
My belief is that the future will be made up of solutions that continuously model and measure incrementality in real-time. Advances in artificial intelligence and data analysis will allow a better understanding of the complex interactions between different marketing channels and isolate the direct impact of campaigns. Predictive models and machine learning algorithms will allow us to anticipate the effects of campaigns and optimize marketing investments in real-time. I have some ideas on this, but I can’t talk about them here. I am in the process of starting a startup on this topic, and I hope to be able to tell you more soon.
In the meantime, if you need help setting up incrementality tests or if you have questions on the subject, do not hesitate to contact me. I work as a consultant in data science, data analytics, and marketing measurement, and I would be delighted to discuss your needs and help you get the most out of your marketing campaigns.
Contact me at franck@lycee.ai or visit my website at www.lycee.ai. I look forward to hearing from you!